Understanding the AI Ecosystem in 2024
2024 may be the year of AI, but the origin story for artificial intelligence as we know it today began several years ago. More precisely, the foundation for the ease with which we all use AI in 2024 was laid by machine learning (ML).
Machine learning dates back to 1959, but the past 10 years have seen data mining and model training make the original goal of machine learning — to advance artificial intelligence — more achievable. A robust, widespread effort to train models for language, imagery and video has presented us with a reality where we employ AI without fully recognizing it.
It's critical that you start to think about your role — and career — differently. This will require a shift toward more soft skills, high-level knowledge of code and codebases, a better understanding of product roadmaps and full-funnel marketing, and a shift toward conceptual thinking and management of AI services. Hard-coding and manual-only processes may not be completely going away, and there will always be a need for tech professionals who can edit and refine code, but these are the types of efforts that are and have been the focus on GenAI so far.
"Above all, a software development professional needs to demonstrate the core skill of problem-solving. When making a long-term hire - someone who will be able to move from project to project and grow - the most important signal is not what technologies they know today, but how they apply that knowledge."
Jason Wodicka, Principal Engineering Advocate at Karat
AI and the Future of Tech Work
AI is not going to take your career, but you will need to adapt. The AI age represents a large shift in how we approach work, and how we can better identify opportunities for increased efficiency and improved accuracy in end products.
Tech professionals must think beyond the rigors of the day-to-day efforts. Instead, it's important to take a high-level approach to the work they have to complete. As AI finds itself in use in more corners of our world, managing it will become a highly prized skill for employers everywhere. Artificial intelligence replaces tasks, not jobs, and there will be an increased focus on results as assistance from AI finds its way to more workflows.
Some AI-based solutions will come in the form of platforms or services that require a subscription, meaning faster implementation and minimal training of models. It's just as likely that tech professionals will have to create solutions bespoke to their needs, including training their own ML models and the results they expect AI to return.
Now is the right time to act and upskill yourself for a future laden with AI. If we think about 2024 and artificial intelligence the same way we consider the early days of the internet, we may find that, in retrospect, getting in on the ground floor of a widespread initiative can be massively beneficial. With AI and its widespread use already happening, familiarizing yourself with how AI and ML work and how to use them in your workflow are critical. As AI has evolved rapidly, so will tech professionals. From AI-driven code completion, we're already seeing fully artificial code being generated. Google Gemini can write code in various languages, and an early model of an artificial intelligence engine that can write more complex code has already been seen.
The question isn't whether AI should generate code, it's how reliable and accurate it is — and that's where humans find their value. We know AI needs a prompt to generate code, and we now understand AI needs the code it generates to be verified, much like code generated by humans does. For resource-constrained teams or engineers who are overwhelmed, AI can be a boon to productivity. It simply requires upskilling.
One thing we are hearing from hiring managers and recruiters right now is that while the skills are critical, they're also looking for tech professionals who are comfortable with adaptability, agility and curiosity. Because GenAI is so new and evolving every day, the skills and applications today may not be the skills and applications of tomorrow. Thus, the ability to change is one of the most important qualities not only in positioning yourself for AI jobs, but for your broader career aspirations as well.
In these early days of widespread artificial intelligence, it's easy to become overwhelmed. Every day brings a new AI model or news of a company leaning into AI more than previously discussed. It's more critical to consider your career, how you can upskill yourself, what skills to focus on, and why it matters to the long-term health of your career.
Where and How is AI Being Used Today?
As you head into the next era of your career, whether you adopt an AI focus or not, it is important for you to have a strong understanding of what sort of projects tech professionals who specialize in AI related skillsets might be working on.
These are just a few examples, and the applications of AI are constantly expanding. With the democratization of GenAI, AI of all forms will likely be inextricably linked with tech jobs and careers.
AI in the Everyday
More Advanced Use Cases for AI
Applications for AI in Science and Industry
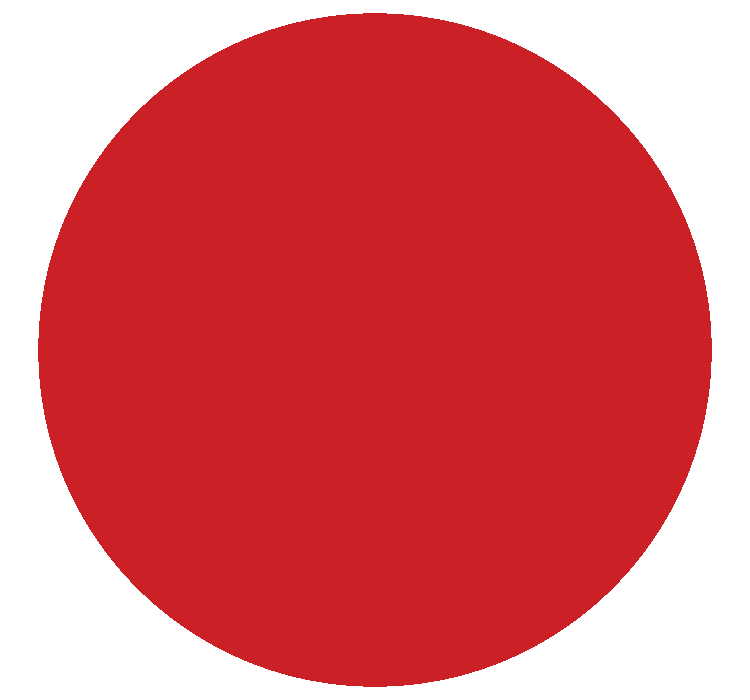
When I look for AI/ML professionals, I look for curious, nimble developers who are always ready to learn about new technologies. Even before generative AI emerged, the skills developers needed were constantly changing; older languages became obsolete as the industry transitioned to the languages best suited for today’s applications. Developers at every point in their career should seek upskilling through online training, experimentation and more, both to obtain necessary skills and to demonstrate their ability to adapt. Change is always hard, but adapting to new tools will lead to huge productivity gains.
Miguel Baltazar, Vice President of Developers at OutSystems
Fitting Generative AI Into the Picture
Generative AI tools such as ChatGPT have sparked a good deal of discussion (and more than a little concern) over the past year. While many professions are awakening to the possibilities of AI generated text and code, the options already available in the market are more varied than most people think.