The desire for
data-science and machine learning (ML) skills will continue strongly into next year, according to developers surveyed by analyst firm SlashData. SlashData queried some 20,500 respondents from 167 countries, which means this is a pretty comprehensive
survey from a global perspective. Responses were additionally weighted in order to “derive a representative distribution for platforms, segments, and types of IoT [projects],” according to the report accompanying the data. According to the survey, some 45 percent of developers want to either learn or improve their existing data science/machine learning skills. This outpaces the desire to learn UI design (33 percent of respondents), cloud native development such as containers (25 percent), project management (24 percent), and DevOps (23 percent). “The analysis of very large datasets is now made possible and, more importantly, affordable to most due to the emergence of cloud computing, open-source data science frameworks and Machine Learning as a Service (MLaaS) platforms,” the report added. “As a result, the interest of the developer community in the field is growing steadily.” In addition, companies such as Google and Microsoft have spent the past few years issuing a variety of developer tools in areas such as computer vision, natural language processing,
and machine-learning models. Not only do those releases help spark developer interest in data science and machine learning, but it also gives them the ability to actually explore and develop new applications. SlashData also found that some 20 percent of machine-learning developers and data scientists are working on projects that involve either image classification, consumer behavior analytics, and/or natural language processing. Even more (26 percent) are figuring out if they want to specialize in a particular area. Machine learning and data science have become intrinsically linked. Just look at this chart, created from Dice data, that shows the vital skills necessary for climbing through the ranks of a data-science career:
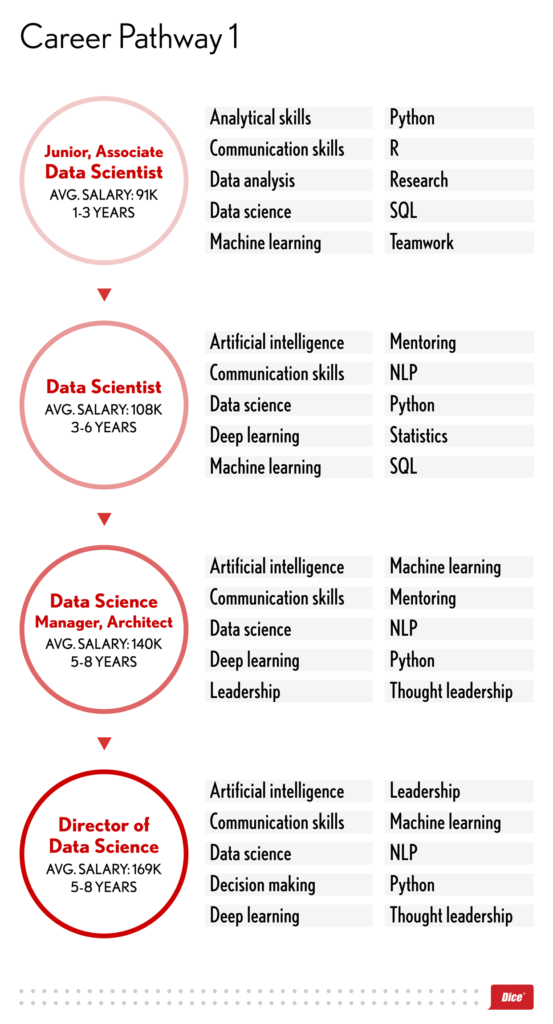
And machine-learning specializations are likewise a key stepping-stone on many a data-science career pathway:
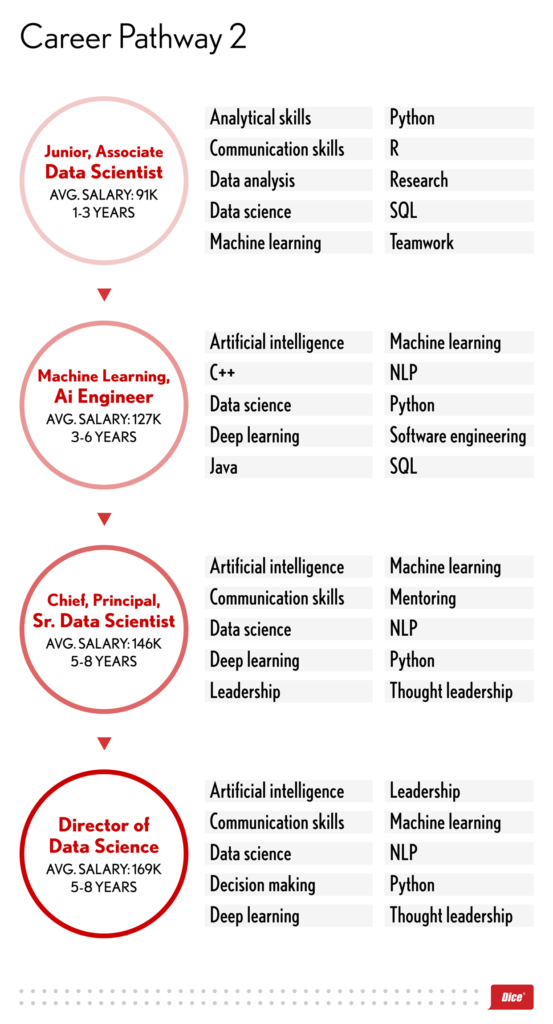
Indeed, the line between “traditional” data scientists and those who focus on machine learning and A.I. is becoming very blurred. For years, data science was a pretty straightforward field: you found or built a dataset that hopefully
wasn’t too noisy, and you used various tools on it to obtain actionable insights. In recent years, though, it’s become increasingly clear that machine-learning models can take those huge datasets and learn how to process them on their own, which means that data scientists need to adopt machine-learning and A.I. skills if they want to stay on the cutting edge of their profession.
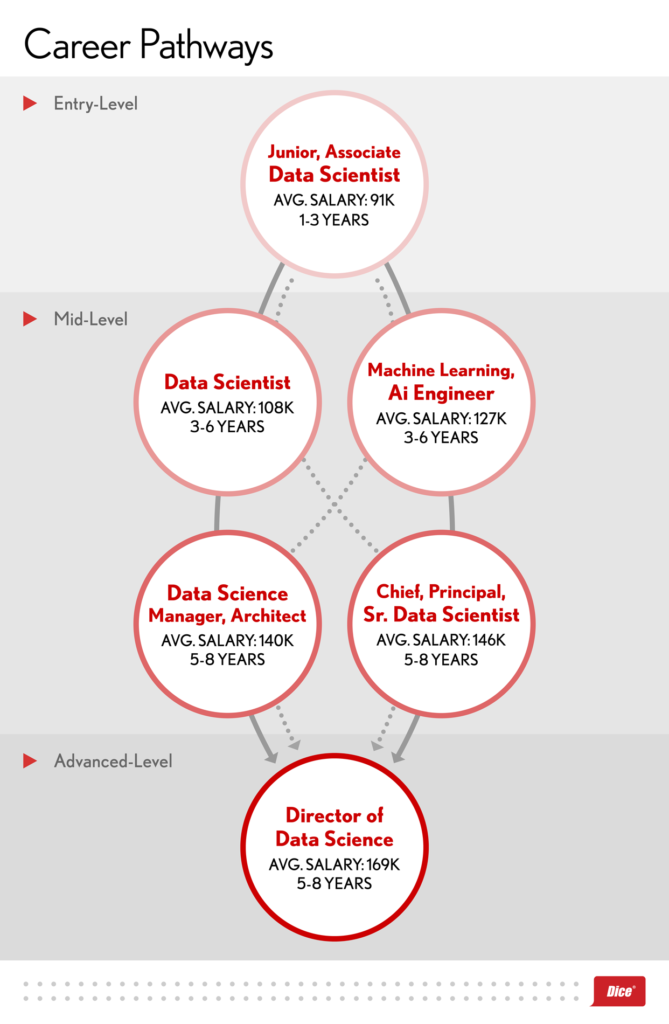
If you’re brand-new to data science, the best thing you can do is learn the Python and R programming languages. While both languages are important to the practice of data science, a recent
KDnuggets poll suggested that the adoption and use of Python has begun to outpace R among data scientists. “Combining R and Python is both reasonable and feasible,” Enriko Aryanto, the CTO and a co-founder of the Redwood City, Calif.-based QuanticMind, a data platform for intelligent marketing,
told Dice earlier this year. “We run them both in our
data science platform internally. But if I were starting my career all over again today, I might consider focusing on Python rather than R. It’s a more-general language with broader applications.” But as machine-learning models perform more analysis on their own, and A.I. platforms synthesize and package data in new and interesting ways, the need to learn advanced ML and A.I. will only grow more intense.